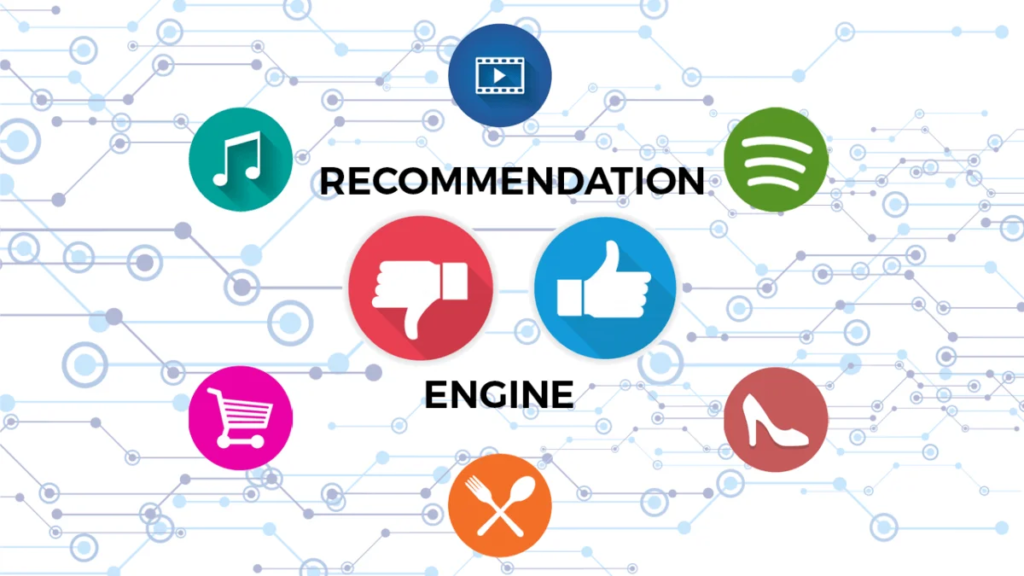
In today’s digital world, recommendation engines play a crucial role in our online experiences. These engines suggest movies on Netflix, products on Amazon, and even friends on social media. But how do they work? Let’s explore the fascinating science behind recommendation engines.
What Are Recommendation Engines?
Recommendation engines are sophisticated algorithms designed to suggest items to users based on various factors. They analyze data to predict what a user might like. Enrolling in a data science course can help you understand the basics of these engines.
Types of Recommendation Engines
There are three typical types of recommendation engines: collaborative filtering, content-based filtering, and hybrid systems. Each type uses different methods to generate recommendations.
Collaborative Filtering
Collaborative filtering is one of the highly famous methods. It works by assessing user behavior and finding similarities between users. For example, if you and another user have similar tastes in movies, the engine will recommend films that the other user likes. Understanding this method in-depth is a key part of any data scientist course in Hyderabad.
Content-Based Filtering
Content-based filtering actively focuses on the attributes of items rather than user interactions. It recommends items that are similar to what a user has liked in the past. For instance, if you enjoy action movies, the engine will suggest more action films based on the characteristics of the movies you have rated highly.
Hybrid Systems
Hybrid systems integrate collaborative and content-based filtering to provide more accurate recommendations. They leverage the strengths of both methods to offer better suggestions. Many advanced recommendation systems, like those used by Netflix and Amazon, employ hybrid techniques.
Data Collection
Data is the backbone of recommendation engines. They collect vast amounts of information from users’ interactions, ratings, and behaviors. This data is then analyzed to identify patterns and preferences. Taking a data science course can teach you how to handle and analyze such large datasets.
Algorithms and Models
Several algorithms and models are used to process the collected data. These include matrix factorization, neural networks, and clustering techniques. Each algorithm has its various strengths and is chosen based on the personalized needs of the recommendation engine. A data scientist course in Hyderabad often covers these algorithms in detail.
Matrix Factorization
Matrix factorization is a technique used to break down large matrices of user-item interactions into smaller, more manageable pieces. This method helps in finding hidden patterns and relationships within the data. It is widely used in collaborative filtering systems.
Neural Networks
Neural networks are used to come up with complex models that can learn from massive datasets. They are particularly helpful in hybrid systems, where both user behavior and item attributes are considered. Neural networks can capture intricate patterns and make highly accurate recommendations.
Clustering Techniques
Clustering involves grouping users or items into clusters based on similarities. This method helps in identifying groups of users with similar preferences, making it easier to generate targeted recommendations. Clustering is a fundamental concept in data science.
Challenges in Building Recommendation Engines
Building effective recommendation engines comes with its challenges. These include data sparsity, scalability, and handling dynamic data. Data sparsity occurs when there are too few interactions or ratings, making it hard to identify patterns. Scalability involves ensuring the engine can handle a growing amount of data and users efficiently. Handling dynamic data means the engine must adapt to changing user preferences in real-time.
Evaluating Recommendation Engines
Evaluating the performance of recommendation engines is essential. Metrics like precision, F1 score, and recall are used to measure their accuracy and effectiveness. These metrics help in fine-tuning the algorithms to improve recommendations continuously.
Future of Recommendation Engines
The future of recommendation engines looks promising with advancements in artificial intelligence and machine learning. These technologies will make recommendations even more personalized and accurate. Continuous learning and enhancement will be key to staying ahead in this field.
Conclusion
Recommendation engines have transformed how we interact with digital platforms. They make our online experiences more personalized and enjoyable. Understanding the science behind these engines requires a solid foundation in data science. Enrolling in a data scientist course in Hyderabad can provide you with the skills and knowledge needed to build and optimize these powerful tools. As technology evolves, so will recommendation engines, continuing to enhance our digital lives.
For More details visit us:
Name: ExcelR – Data Science, Data Analytics and Business Analyst Course Training in Hyderabad
Address: Cyber Towers, PHASE-2, 5th Floor, Quadrant-2, HITEC City, Hyderabad, Telangana 500081
Phone: 096321 56744
Direction: https://maps.app.goo.gl/sqeQGqodXvjH5b2H6